The Energy Efficiency Benefits of Shifting Intelligence Gradients Upwards
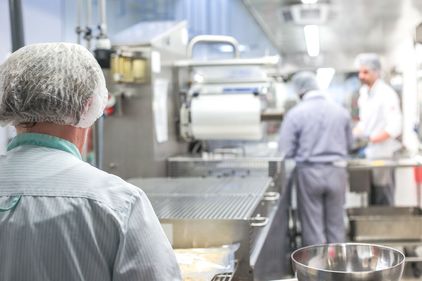
The Energy Efficiency Benefits of Shifting Intelligence Gradients Upwards
Gradients are temporary states of disequilibrium, and they are what makes the world go round. Temperature, pressure and gravitational gradients drive climate, and these and other gradients such as electrical charge drive engineered systems; chemical gradients drive physiological functions and chemical reactions; supply-demand gradients drive economies and trade.
Within economies, ownership is a critical determinant of gradients. Ownership of real estate corners part of a market; ownership of intellectual property creates exclusivity of supply. The same also applies to data – except Big Data. The big idea with Big Data is that it isn’t exclusive, but is a pre-competitive resource which competing actors can use to create their own gradients. These are in the form of distinct and profitable contributions to marketplaces which are usually associated with the common good because most people need the goods and services involved. The Bigness of the data is because there are so many generators or contributors to it, or it represents many events or entities. Big Data is defined by the three Vs – volume, velocity and variety.
In SMART’s field of sustainable resource use in manufacturing, Big Data hasn’t really arrived. In the UK, through the annual PRODCOM Survey, we know about the types and amounts of energy used by whole industry sectors, but this is not broken down into sub-sectors or stages of manufacturing in each sector; there is no common information below the economy level about materials, water and chemicals flows and conversions. For manufacturing resource use, there is really only Small Data, kept by individual companies and in some cases their software providers, and No Data, where even a company does not know about some of its resource use.
This problem of Small Data has been highlighted in reports such as the Made Smarter Review 2017, led by Siemens’ CEO Juergen Maier. This endorsed the idea of data trusts – proven and trusted frameworks and agreements – “to overcome one of the biggest inhibitors in exploiting industrial data technologies in manufacturing: a reluctance to share data”.
One particular set of manufacturing data which could usefully become Big is the energy use profiles of machines for defined tasks or product outputs. This has been extensively researched for machine tools, mainly the working of metals, and the public data pool is in the form of many academic papers. More recently at SMART, we have been researching energy use in food manufacturing. An easy initial observation was that well-known improvements to the operation of existing machinery and management actions are gradually being implemented. Beyond this, we also found that there is plenty of potential for reducing energy use through technical and process innovation, but that the use of that potential is being determined by the Small Data which currently represents it. Comparable machine energy data is low in volume, velocity and variety, hardly being generated or gathered. As a result, we reckon that the innovation activity is far lower than it could be.
A major conclusion from the research is therefore that comparable machine energy data needs the Big Data treatment. Imagine a virtual resource holding scientifically valid and robust comparisons of energy use by different machine designs, embodied in brands, making the same product. This would be a pre-competitive resource which actors in the food manufacturing ecosystem could use to add their particular value to the energy efficiency of production. Visibility would be a key attribute, much as a mirror or a video is an effective instructional aid. The machinery manufacturers would be able to see how their products perform against peers; software developers would have much bigger and richer datasets on which to perform useful analytics, compared to the datasets from their limited pool of clients; energy consultants could take their insights to a more sophisticated level; engineering consultants could innovate with the prospect of a much wider impact than single client contracts can offer; and food manufacturers would see the scope for improvement through their anonymised place in energy league tables for the products in question. Peer psychology would operate to stimulate attention to improvement, as has happened in other sectors. The availability of a richer pool of energy data would free resources for competitive aspects of the food business such as product performance and marketing.
Other users of this Big Data for the common good would be academics, government departments and agencies and utilities producing power and fuels.
SMART is hoping to stimulate the creation of such a resource for food and other manufacturing; a large reservoir of Big Data from which gradients of intelligence at a higher level can be generated, in order to drive accelerated energy efficiency improvements and reduce the environmental and financial cost of all our food products.